Predictive analytics uses different sets of data and techniques to answer the question: what is likely to happen in the future based on historical and current data? The same can also be used to determine what can be done about future events.
Organizations obtain such insights using predictive models in predictive analysis software. They are capable of doing so because, under the hood, they have powerful technologies and techniques. These are the ones that enable you to gain valuable insights into the future so that you could make intelligent decisions regarding the growth of your company. Below are only a few examples of technologies and techniques that help you prepare for the future.
- Artificial Intelligence and Machine Learning
According to a SmartData Collective article, globally, people are generating more data currently than they have in the past years. In fact, the current amount of data we have worldwide was generated only between 2016 to 2018, with 2.5 quintillion bytes of data produced per day during the period.
And yet, despite this huge amount of data, some companies manage to sift through so much data to find valuable and actionable insights. This is possible because of artificial intelligence (AI) and machine learning (ML).
But what are these?
First, artificial intelligence is intelligence demonstrated by machines, which is why it is otherwise called machine intelligence. It simulates some of the mental functions of humans such as thinking, learning, and analysis. In predictive analytics, we use AI to process large volumes of data. It can even accurately categorize information when it acquires enough data and has learned from them.
That is where machine learning comes in. It can read datasets to find patterns in them. ML then applies what it has ‘learned’ to improve its knowledge and provide better and more to-the-point results in the future. This means that as you continue to use a system with a machine learning feature, it can continuously learn from your data and actions to enhance the service. And in predictive modeling, it uses pattern recognition to predict future events.
To put it in perspective, here is a predictive analytics example, albeit a simplistic one: setting up customer support chatbot that can accurately determine the issue a customer wants answers for. The chatbot is provided training data that comes from the historical and current data of a company, such as chat conversations between clients and customer service representatives. From it, it is able to detect patterns by reading words, phrases, and the like, which it models again to learn from it (this process is continuous). And so in the next interaction with a customer, it can predict what that customer wants and provides solutions to them without further ado.
- Geographic Information Systems
Did you know that Geographic Information Systems (GIS) can also be helpful in predicting the success of a company or a campaign in a certain area? It can work when it is connected with predictive modeling tools (more about connections later).
Let us discuss what is GIS first.
It is commonly used in gathering data and mapping it. It is rooted in geography and is being used in different fields such as sales and marketing for predictive analysis in the success of a store in relation to the environment.
It can support multiple layers of data such as the weather during a certain period, the number of people that went into shops, and how many of them actually converted. By analyzing these datasets, it becomes possible to predict the future movements of people depending on the weather, which allows stores to make strategic decisions such as closing early or extending their opening hours.
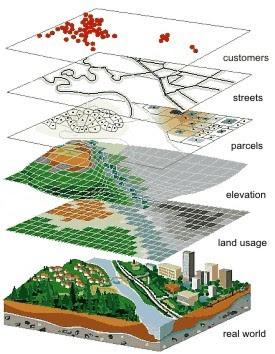
Thus, retailers can maximize even weather data when it comes to pushing certain items of clothing to customers.
- Integrations
Earlier, we mentioned connections. Those are essential to gathering different types of data for predictive analysis to be more accurate. They can come in the form of APIs, connectors within applications, or made possible through integration solutions.
Most software programs developed nowadays have API or allows synchronization via an integration platform. With this, it can exchange data with other systems. A common use of this function is for data entry, especially when interfaces require the same or similar set of data.
It has its uses in predictive analytics, too. Since integrated software can draw and provide information, it is possible for the predictive analysis application to have complete or more comprehensive historical and current data. This way, it can produce in-depth and accurate predictions.
Without integrations, people would have to manually feed data into the analytics software, which is both time-consuming and prone to human error.
Preparing for the Future
Predictive analytics are essential to the survival of a company. Just the sales data alone can have great impact on the future of a business. But coupled with geospatial information, it is possible to predict even the behavior of consumers during different weather conditions and other environment-related scenarios.
With this, the intelligence of decision-makers is compounded, enabling them to make choices that are backed up by numerous types of data. Plus, it allows them to take advantage of the environment or setting their store is on for promotions and campaigns to attract more buyers.
If you’re interested in a GeoSpatial AI Business Intelligence tool, you can check out Fract Territory. For more information, you can also book a free 30-minute session with its GeoSpatial Intelligence experts.